Here in the second of my AI article series for LinkedIn, I explore the rapid pace of AI development, the influence of converging technologies and AI’s trajectory in the short, medium and long term.
When 2 or more technologies merge, it can create a powerful synergy, and the potential for truly world changing products. Just try and imagine life before the iPhone? Here’s 3 technology pairs that converged to amplify its product impact:
Touchscreen and Mobile Computing – Integrated capacitive touchscreen with mobile computing for intuitive interaction and a seamless user experience.
Telecommunications and Internet – Merged cellular technology with internet access, transforming the iPhone into a phone and a web portal.
Digital Photography and Mobile Devices – The combination of a high-quality camera and image processing software has totally revolutionised mobile photography, proliferating communications and ushering in a completely new social paradigm.
With every major tech firm globally, plus a crop of new unicorns, this break-neck development pace is going to accelerate even more rapidly – like Moore’s Law on steroids. Below’s graph shows how compute power has increased since 1970.
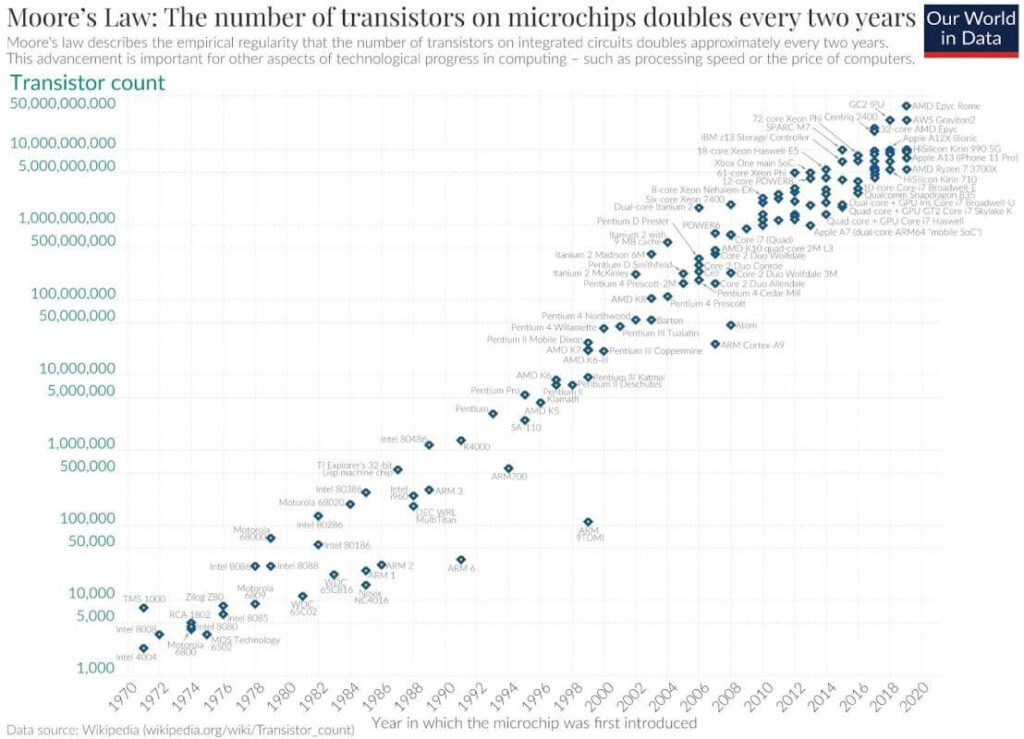
Now, if you observe the same phenomena in AI, and replace transistor numbers with Petaflops, we get the graph below:
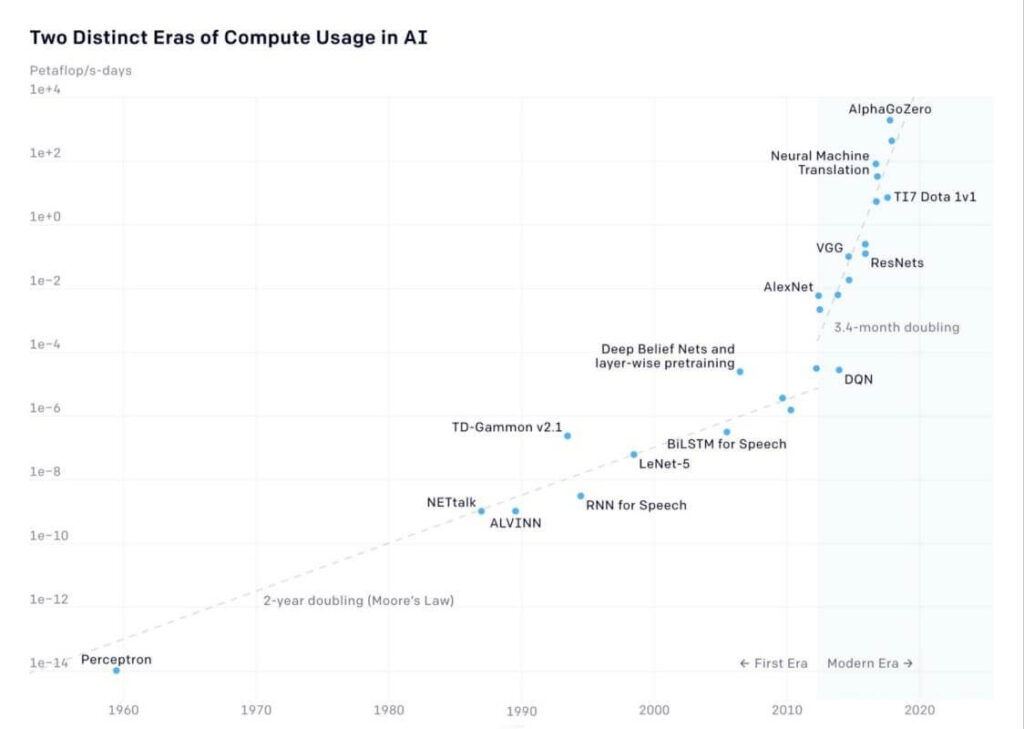
Since 2012, defined as the ‘Modern Era’, AI technology has enjoyed a significant increase of development pace in compute performance. Although the recent era has seen incredibly rapid progress, the impact of AI based technology is going to ramp up again in the next few years.
Key Technology Advances since 2012
Increased Computational Power – More powerful and efficient hardware, specifically advanced GPUs, has allowed for vastly faster and more complex computations. This allows us to train significantly larger and more capable models.
Advancements in Algorithms and Models – Continuous improvements to machine learning algorithms, especially neural networks within deep learning, natural language processing (NLP) and transformers have underpinned the most recent LLMs (Large Language Models) as we see in the likes of Chat GPT.
The Explosion of Data – Global data is increasing in volume at an unprecedented rate. This provides the valuable fuel for training more advanced AI models, allowing them to learn from broader, more diverse information. Context below:
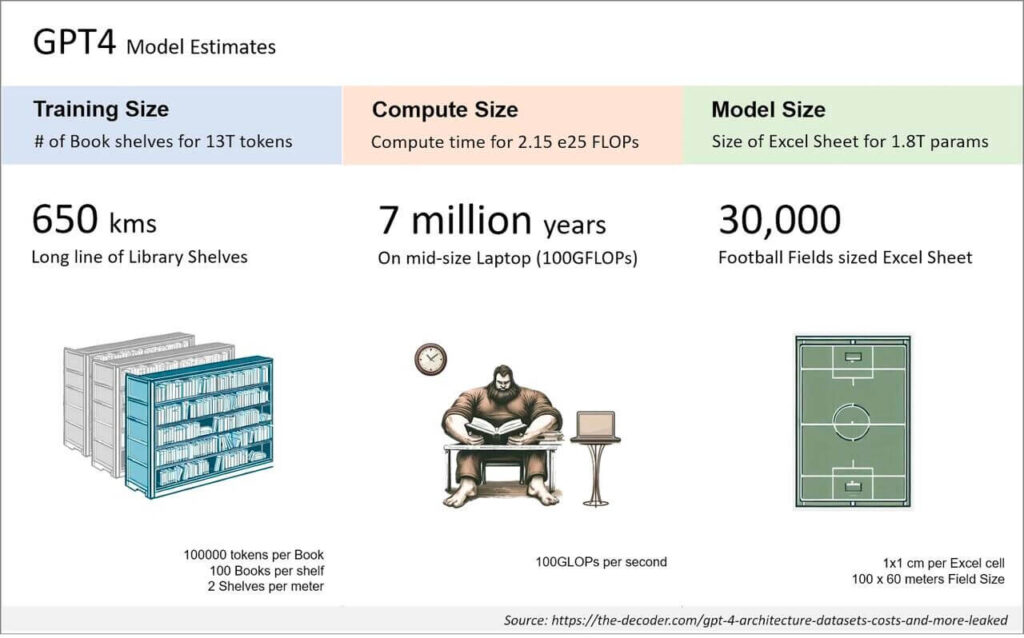
Investment – The prize to gain the upper hand in AI capability is commercially unprecedented. The promise of massive ROI has led to venture capitalists, private investors and companies alike ploughing vast sums of capital into AI and the supporting tech – giving some environments an open cheque book. Just consider this investment in compute power recently by Meta’s Mark Zuckerberg where he’s spending $10bn on 350,000 high end Nvidia graphics processing units (GPU).
If Artificial General Intelligence is achieved – and that definition is open to interpretation – the owner of that IP will undoubtedly have the keys to affect every area of commerce globally, and some say, humankind. For this reason, the investments in AI are being positioned as existential for the larger tech companies.
Tech Community Collaboration – The AI community has very effectively leveraged collaboration and open-source initiatives. Open-source tools and platforms may lower the barrier to entry, allowing more researchers and developers to contribute to AI’s progress outside of the walled development environments usually created by companies.
Commercial and Practical Applications – As the ROI against development cost has been justified, the demand for AI solutions across various industries has surged. Businesses are rapidly expanding the scope of AI embedded platforms, from enhancing customer experience to optimising operations. This success is driving the cumulative effect of development and efficiencies, thus increasing budgets for yearly AI spend.
Global AI Talent Pool Growth – The number of professionals specialising in AI has grown, contributing to a more robust and innovative field. Education and training in AI have become more accessible, expanding the pool of talent contributing to advancements in AI.
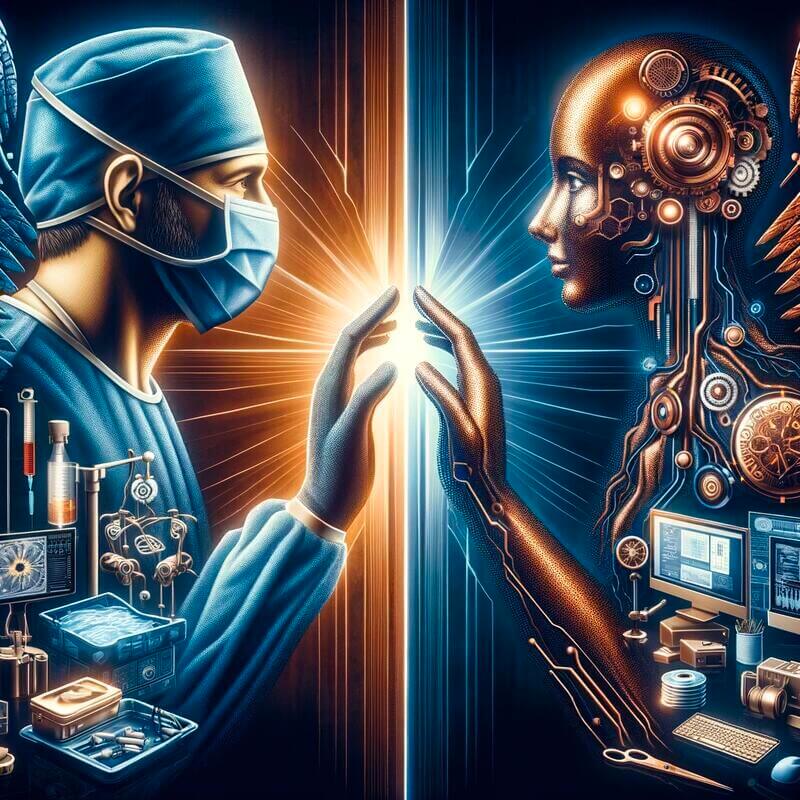
Technology Convergence
When we merge 2 or more of these fast improving AI focussed technologies the synergies can be greater than the sum of their parts. Here are 4 powerful examples:
Natural Language Processing (NLP) + Computer Vision (CV)
Medical Image Diagnosis – NLP algorithms can extract information from medical records and patient history, while CV analyses medical images (X-rays, MRIs, CT scans). Integration here empowers healthcare providers to create a more comprehensive patient profile, allowing better diagnostic accuracy and personalised treatment plans. This synergy improves the overall process, speed and healthcare outcomes, especially in areas like radiology.
Reinforcement Learning (RL) + Generative Adversarial Networks (GANs)
Autonomous Robotics – RL enables robots to learn and adapt to their environment through trial and error, while GANs can generate realistic and diverse data, including images. When combined, RL-driven robots can leverage GAN-generated data to simulate various scenarios for training and testing. This synergy is particularly useful in the development of autonomous vehicles and drones, helping them navigate complex real-world environments more effectively.
Speech Recognition + Sentiment Analysis
Customer Service Agents – Integrating speech recognition with sentiment analysis enables agents (not to be confused with more simplistic, rules based chatbots) to not only understand spoken language but also gauge the emotional tone of the conversation and adjust their responses accordingly.
This synergy is invaluable in improving customer service experiences. For example, an agent can detect frustration in a customer’s voice and respond with empathy, leading to better customer satisfaction and issue resolution. This area of AI will play a key role in commerce due to its scalable effectiveness and the ability to generate additional lead opportunities without employing more staff, whilst presenting a professional image of the company they are employed by.
Internet of Things (IoT), 5G and Edge Computing
Smart Cities – OK, so I cheated, this is 3 things, but it’s worth mentioning the combination. IoT devices within a 5G network plus edge computing capability is a very powerful combination. Consider the amount of data gathered using multiple technologies in the Smart Cities of the future. The inherent low latency of 5G is well suited to focussed edge computing employed by state of the art IoT devices distributed across wide areas. Huge efficiency gains will be made by shifting cloud processing to local devices.
Now, imagine that you can combine any number of technologies – maybe ones that are currently siloed in your company – and you suddenly have a vast number of opportunities to make your commercial processes more efficient and create completely new business opportunities. To ensure you have the best chance of success you need to take an AI approach. It’s vital to approach this novel AI paradigm with a strategy to avoid unnecessary wasted efforts at the same time as recognising you will not get it right, first time, every time.
It’s refreshing to know at this stage the existing strategy to ‘fail fast, learn fast’ still applies. This will be covered more in future articles along with the critical importance of companies maintaining their subject matter expertise throughout. As good as AI is, it’s far from being in a position to take the place of our most valuable asset – people.
The flipside of all this potential is the critical need for greater efficiency. As AI use is scaled and integrated across all business operations, we will hugely increase demand on the associated energy costs and drain the finite global compute resources, so efficient design and operational strategy is going to be a critical factor. Already the early adopters, such as myself, have usage limits placed on GPTs where you are locked out of the systems if you submit too many questions within a short period of time.
Future Game Changers
If you have followed AI news in the last year, you’ll be aware of the daily advances and new products from numerous companies and social media AI commentators alike. I’ll say this throughout my articles though, be careful where you read your news. Remember, YouTube commentators, as informed as they may be, are in the business of ‘like & subscribe’ and not so often in the business of ensuring AI solutions create a meaningful ROI.
The timeline on future AI progression is a subject for huge debate, but the dates are so affected by the opinions of those with vested interests in outcomes to suit their own narrative. As of January 2024 it’s almost impossible to filter out the bias and create a clear prediction beyond this year.
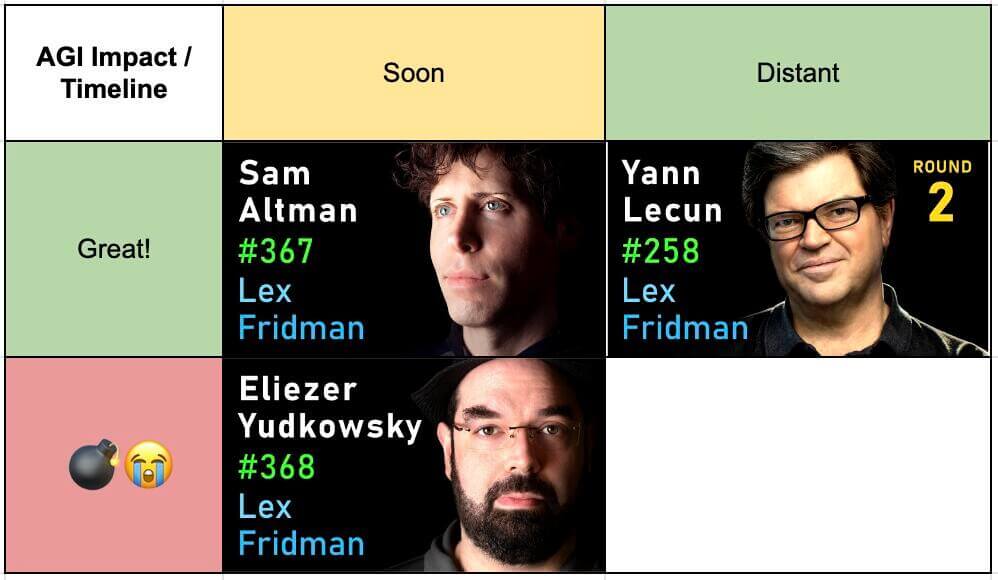
Achieving Artificial General Intelligence (AGI) – Seen equally as the saviour or the death knell for mankind. AGI is an advanced state for AI possessing the ability to understand, learn, and apply intelligence across a wide range of tasks, similar to a human. This milestone, widely considered the ‘Holy Grail’ of AI, would undoubtedly unleash enormous change with AGI applications being able to learn effectively with little or no supervision.
While the visual above lacks dates for AGI to land, it’s useful to see which leaders think it will be sooner and whether they feel that’s a good thing or not! All we should consider at this stage is that AGI is predicted to be achieved anytime between late 2024 and some time in the 2100’s – depending also, on what the true definition of AGI represents.
Ethical AI and Regulation Frameworks – While not a technical advance, per se, this is surely a critical body of work required to safeguard the world against unbridled ‘progress’. Regulations to protect individuals and control the activities of potential bad-actors, privacy, bias, transparency, and accountability are not yet in place, despite a recent lengthy US Executive Order being announced at the November 2023 AI Safety Summit, in Bletchley, UK.
GDPR already covers explicit permissive use of personal data and will be strengthened by the proposed EU AI Regulation but not forecast before 2026 – which is a long time in AI! Concerns are already being pushed into the courts with recent litigation over the use of content within OpenAI’s LLMs by the New York Times.
Quantum Computing Integration with AI – One of science’s ‘Holy Grails’. The compute power of quantum computing makes today’s supercomputers look like slide rules. Combining quantum computing with AI (QAI) could bring solutions for the most complex and seemingly impossible data and computation intensive fields of drug discovery, materials science and weather systems, within realistic reach.
For context, a quantum computing task taking 4-5 minutes would take today’s most powerful supercomputer 10,000 years or more to complete. But… and this is a big caveat, quantum computing is far from a commercial reality. Current issues are numerous, complex and costly to resolve. They include quantum decoherence where calculations are destabilised by noise from vibration and radio waves plus error correction issues created by the inherent instability of quantum computing compared to traditional transistors. See this Silicon Republic article for more quantum wizardry.
Conclusions
Converging technologies supercharging AI potential with a cumulative effect. Much like the rock pools at the top of the article, once the technology tide washes over them, the possibilities are virtually endless.
I also believe that in AGI, the commercial world is pursuing the greatest commercial prize that has ever been known.
I think AGI, or a form of it, will be achieved. I don’t believe it will be a binary event though and like all our LLMs today, it will require fine tuning over a many years. The clamour to be the company who achieves AGI first, will lead to premature claims only to be contested by rivals and, rightly so.
Any major AI advances must be subject to heavy regulatory scrutiny before being made available as this is the only way we can manage this tech that’s developing way faster than the regulatory framework around it. A healthy combination of open source and proprietary development environments should also be maintained to decrease the risk of monopolisation.
I also firmly believe that the transition to a new AI powered workplace will be gradual and require every incumbent subject matter expert to design and orchestrate the transformation. So while there will be significant job displacement, new roles will be developed and augmented roles should become less arduous and more productive. Which I’ll cover in my next article…
If you have found this useful and would like to know how I might help your company, please reach out to me.